What is a Deep Fake?
A Deep Fake is a video in which fake images are shown, usually of a person’s face, which appear to be real and have been produced using Artificial Intelligence. Specifically, Machine Learning techniques called Deep Learning, deep learning, which uses neural network algorithms.
Falsification and alteration of photos is nothing new, however, Artificial Intelligence makes it possible not only to alter images, but to create them. Until a few years ago, it was so expensive to perform a face swap on video clips that only a few movie studios could afford the hundreds of thousands or millions of euros it cost.
Nowadays, technology has advanced a lot, being much more accessible so that anyone can make a “Deep Fake”, with greater or lesser quality.
It is extremely relevant to highlight two aspects, verisimilitude and purpose. The first “Deep Fakes” had rather mediocre and not very credible results. As advances have been made in Machine Learning, the results are amazing and increasingly difficult to detect. On the other hand, there is the intention with which the video was created. It is not the same to use it for scientific purposes or to create authorized content, than to generate false information. The law does not regulate the use of technology, but it does regulate the purpose for which it is used.
How is a Deep Fake created?
Artificial Intelligence recreates an image of a face or any other object by learning from hundreds of thousands of images of that face or object. It uses what are called generative antagonistic neural networks, GANs, with algorithms that are able to learn from the patterns found in the images, and then reproduce them by creating new ones of that object, face or image.
In 2017 researchers at the University of Washington used more than 14 hours of recordings of President Barack Obama to reproduce his image and voice to simulate any speech. They created a model of the shape and movements of the mouth to link it with the voice recordings. With this technique, on real videos, they could put into Barack Obama’s mouth any message that an actor reproduced.
In 2018 a group of professionals made a Deep Fake, not very good, but funny, of Texas Senator Ted Cruz singing and imitating Tina Turner. In this case, the algorithm model encoded how the senator’s face gesticulates, moves and looks and that of an actor. It then decodes the images of Ted Cruz’s face and reconstructs them over those of the actor’s face.
In short, Deep Fakes work through the use of generative neural network models, Deep Learning. Basically, the algorithms learn to create images of real or fictitious people after processing a database of example images. From being trained with images of a specific person, they can generate very realistic videos of that person. In a similar way, voice is recreated, with the potential for both positive and malicious use, creating totally believable fake videos of people doing or saying something inappropriate.
The plausibility of these reconstructions is complicated if the images on which the model has learned differ greatly from those to be linked. Sometimes results are produced with ears, noses, or jocular features.
The most popular Deep Fakes are of celebrities as there is an immense amount of photos and videos available on-line, but you can do the same with anyone as long as you can get enough images, for example, from social networks.
There are several applications and solutions for creating Deep Fakes. Computer hardware with very powerful graphics processors is required, as image processing can take even days for a few minutes of video. However, this can be accelerated with the use of virtual machines available on multiple cloud platforms.
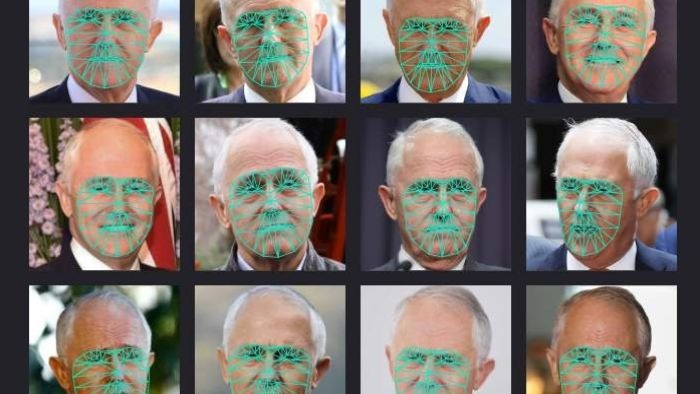
What were the first Deep Fakes?
In 2018, its use in videos with sexual content began to be heard frequently, although the innovation started in 2014.
In 2017 an anonymous Reddit user used Deep Learning, to swap the faces of famous actresses with those of the original actresses, in adult movie scenes.
In 2014 Ian Goodfellow, a PhD student at the University of Montreal, tackled image generation in a pioneering way with the generative adversarial neural network approach, GAN. Goodfellow trained two neural networks with the same image database and then created new ones. He pitted the two networks against each other to identify which images were real and which were fictitious, like a digital cat-and-mouse game.
The first neural network model generated new images from the database it had learned, creating for example a cat with two tails. The second model detected the fictitious images, and so the first model learned from its own mistakes and generated cats with only one tail. Gradually, more and more realistic and difficult to detect images were created.
These AI techniques have been used by research teams to create fictitious faces from celebrities, or to create paintings supposedly by Van Goh.
Originally, these neural networks made a lot of mistakes, such as bicycles with two handlebars, or faces with eyebrows out of place. Now they are able to create with high plausibility a complete image from a part of it, for example, the body of a cat from its head.
Malicious uses of Deep Fakes?
This Artificial Intelligence technology, unfortunately, can be used maliciously to deceive governments, populations, cause international conflicts, damage a person’s image, or take illegitimate advantage.
It all started in adult movies
In 2017 Deep Fakes with celebrities began. Especially popular were the fakes of Emma Watson and Natalie Portman. Video clips have also been made of former First Lady Michelle Obama; former President Donald Trump’s daughter Ivanka Trump; or the Duchess of Cambridge, Kate Middleton.
Unfortunately, politics has tried to take advantage of the
At the end of 2018, Gabon’s President Ali Bongo had not appeared in public for months, and his state of health was beginning to be questioned. To quell the rumors, a video was released in which he delivered his usual New Year’s speech, with the peculiarity that he did not blink in the more than 3 minutes that the speech lasted. For verisimilitude, details are important.
This past U.S. election campaign, Deep Fakes posed a risk to politics in terms of fake media appearing to be real.
Speaker of the House Nancy Pelosi, has come under multiple attacks. A recording of an interview was altered to make it look like she was drunk. These images were posted on social networks and were shared more than 45,000 times and had more than 23,000 comments alluding to her apparent drunkenness.
In September 2020, fake versions of Russian President Vladimir Putin and North Korean leader Kim Jong-unveiled the same message, that he did not need to interfere in the elections as it would be the United States itself that would ruin its democracy by itself.
Not everything is manipulation, nor does it all happen in the United States. In February 2020, a few days before the state elections in Delhi, a video of Manoj Tiwaroi, president of the Bharatiya Party, went viral in India. In the original video he speaks in English criticizing his political opponent. Whereas, in the viral video, Artificial Intelligence has been used to make him convincingly move his mouth while speaking in the Hindi dialect used by most of the targeted voters.
Impersonating to defraud
A highly credible Deep Fake audio impersonation of the voice of the CEO of a UK energy company, asking a managing director to make a transfer of €200,000 to a supposed Hungarian supplier, was highly publicized. By the time the scam was realized, the money was already scattered around the world having been moved through accounts in Hungary and Mexico.
Positive uses of Deep Fakes?
Technology is harmless in itself, a good use can have a great positive impact on people’s lives, businesses and society. The legitimate use of the image and voice of third parties opens up great business opportunities in the world of television, film, marketing, etc.
Artificial Intelligence in documentaries and journalism
JFK’s words in July 1963 ushered in the resolution to end the Cold War. His assassination on November 22 of that year changed the pace of history, causing upheaval around the world, and his speech at the Dallas Trade Mart was never heard. In 2018, that speech was heard in the recreated voice of JFK himself, thanks to an initiative of the Irish company Rothco. Using Artificial Intelligence and over 8 weeks, recordings of 831 speeches were analyzed and the voice was constructed by dividing it into 116,777 small phonetic units. The biggest challenge was to capture the speech style and the difference in quality of the recordings from different dates and recording equipment. This was the first speech made entirely using Artificial Intelligence.
A similar approach was taken by two MIT researchers, Francesca Panetta and Halsey Burgund, in the event that the 1969 Apollo lunar landing was a disaster. President Nixon had two speeches prepared, in the event of the successful, or unsuccessful, completion of the moon adventure. The MIT researchers followed the same steps as in the case of JFK, and used actor Lewis D. Wheeler as the basis for superimposing the President’s image and voice. It took days in the lab to train the Deep Learning algorithms to link the actor’s voice and face to Nixon’s.
In June 2020, Welcome to Chechyna, an investigative film about the persecution of LGBT people in the Russian republic, became the first documentary to use Deep Fakes to protect the identities of the people involved from persecution. Volunteer LGBT activists from around the world were asked to lend their faces to be impersonated by 23 of the film’s protagonists.
More recently, Reuters used Artificial Intelligence to recreate news reports from real journalists, and almost in real time, depending on the events and without requiring the recording in person.
Deep Fakes in television and movies
Last year, an advertisement was made in the United States to promote the return to active professional sports. The ad starred NBA player Damian Lillard, WNBA player Skyler Diggins and field hockey player Sidney Crosby. None of them went to the recording studios; it was actors who made the advert, using the faces and voices of the athletes.
Soccer player David Beckham starred in a campaign against malaria. He recorded a single video clip on which Artificial Intelligence was applied to play the same message in nine languages. His facial movements were manipulated, creating the visual illusion that he was actually speaking in each language, and even a female voice.
This year, the Cruzcampo ad with the Deep Fake of Lola Flores has been all the rage. More than 5000 images of “La Faraona” have been used to link her face and voice with that of an artist who represents her.
Culture and education also exploit Deep Learning
The Salvador Dalí museum in St. Petersburg, USA, has recreated the image and voice of Dalí. He interacts with visitors and even takes a selfie with them. Its creation required more than 6,000 frames and 1,000 hours of machine learning. His facial expressions were linked to those of an actor with body proportions similar to Dali’s, and the voice was synchronized to mimic his unique accent, a mix of French, Spanish and English.
Medicine has long been using these Artificial Intelligence techniques.
Generative adversarial neural networks (GANs) are used to create digital twins, and create new images of brain tumors by changing their location and size, or images of skin lesions or liver lesions. With these new images, Machine Learning models can be trained when a base of real images is not as large as desired.
These same Deep Learning techniques are used for cancer detection. The algorithms learn from a large database of radiology images that have previously been labeled with presence or absence of tumors. From here, the AI solution is able to identify evidence of tumors in a new image.
The future of Deep Fakes
There is no doubt that the Artificial Intelligence technology behind Deep Fakes, Machine Learning, has a present that is already very promising.
As progress is made in Deep Learning techniques, neural networks, the range of possibilities will grow rapidly, in different fields, such as health, education or business.
However, as access to this technology advances and increases, so does the risk of Deep Fakes being used for malicious purposes.
Artificial Intelligence itself can be used to detect Deep Fakes. Companies like Google have launched a database with thousands of manipulated videos to develop tools to detect fakes.
This cat-and-mouse game of creating counterfeits and detecting them is actually accelerating innovation in this field, which must serve a positive use.
Andrés Visús, Head of Business Development at PredictLand