As a data scientist at PredictLand, Eduardo Sánchez Burillo, has carried out a research on the application of machine learning on symmetries in Physics, jointly with CSIC (Consejo Superior de Investigaciones Científicas) and the University of Zaragoza. It was published last week in Arxiv, and sent to other scientific journals for peer review.
Under the title “Finding discrete symmetry groups via Machine Learning”, the study introduces a new approach based on machine learning, called Symmetry Seeker Neural Network. This method, without requiring prior knowledge of system symmetry or mathematical relationships between parameters and properties, can identify the finite set of parameter transformations that preserve the physical properties of the system. Its versatility is demonstrated using examples from mathematics, nanophotonics and quantum chemistry, marking a significant milestone in the understanding and application of artificial intelligence in science.
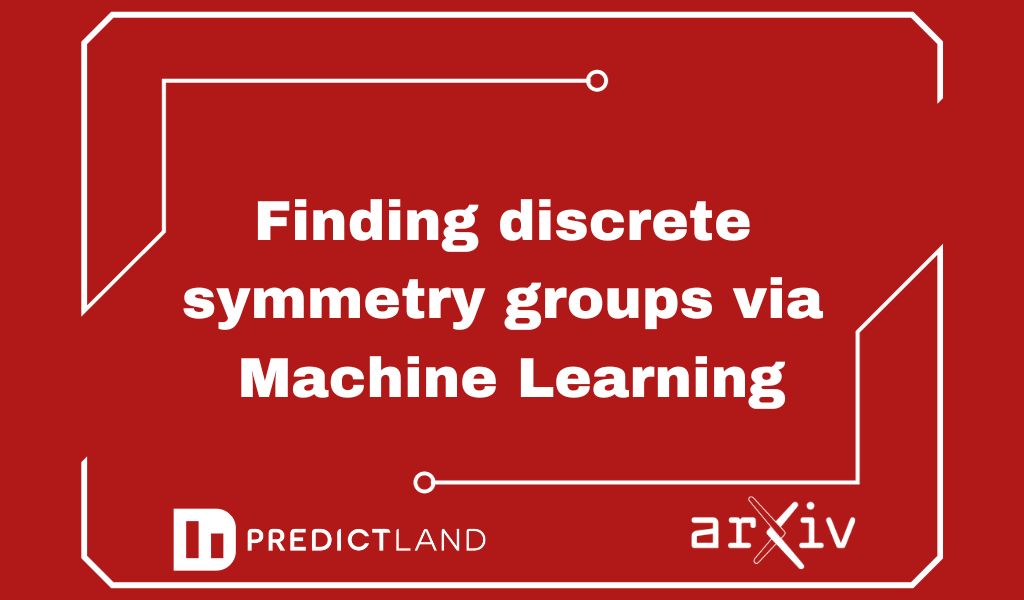